Find out how Cornell’s CAST is changing dairy farming with new technology. Can sensors and AI make cows healthier and farms more efficient?
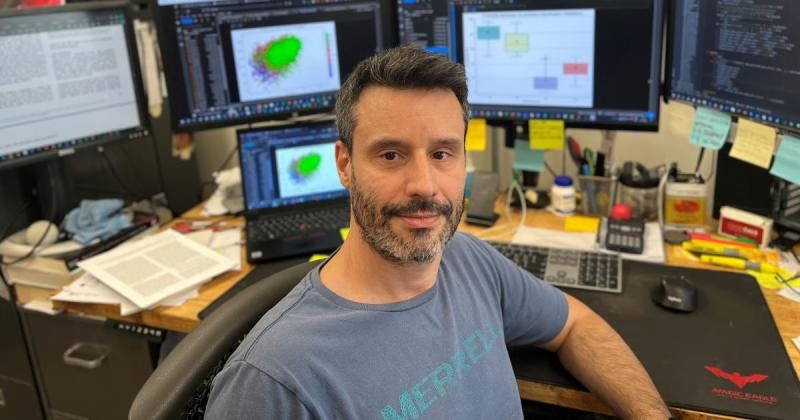
Imagine a day when dairy farming effortlessly combines with cutting-edge technology to enable autonomous systems and real-time herd monitoring using data analytics. Cornell University’s CAST for the Farm of the Future is helping this vision. Under the direction of Dr. Julio Giordano, the initiative is using environmental monitoring, predictive analytics, autonomous vehicles, and livestock sensors. Promising detection of diseases, including mastitis, enhancement of cow health, and increased farm efficiency have come from automated systems evaluated. Many sensor streams—tracking rumination, activity, body temperature, and eating behavior—are examined using machine learning algorithms for proactive health management. Other CAST efforts promote optimal nutrition and feeding as well as reproductive surveillance. Globally, food security and sustainable, practical farming depend on these developments. Offering scalable solutions for contemporary agricultural demands and a more sustainable future, CAST’s work might transform the dairy sector.
Revolutionizing Dairy Farming: Cornell’s CAST Paves the Way for Future Agricultural Innovations
The Cornell Agricultural Systems Testbed and Demonstration Site (CAST) is leading the modernization of dairy farming with innovative technologies. Establishing the dairy barn of the future, this project combines digital innovation with conventional agricultural methods. CAST builds a framework for data integration and traceability throughout the dairy supply chain through cow sensors, predictive analytics, autonomous equipment, and environmental monitoring.
CAST gains from. The Cornell Teaching Dairy Barn in Ithaca and the Musgrave Research Farm in Aurora are three New York locations. Every area is essential; Harford emphasizes ruminant health, Aurora on agricultural management and sustainability, and Ithaca on education and research.
These facilities, taken together, provide a whole ecosystem that tests and shows agricultural innovations while training the next generation of farmers and scientists. Through data-driven choices and automation, CAST’s developments in dairy farming technologies aim to improve efficiency, sustainability, and animal welfare.
Leadership and Vision: Pioneers Driving Innovation in Dairy Farming
Dr. Julio Giordano, an Associate Professor of Animal Science at Cornell University, is the driving force behind the Cornell Agricultural Systems Testbed and Demonstration Site (CAST). With his extensive knowledge and experience, Dr. Giordano is leading the effort to integrate cutting-edge technologies into dairy production, focusing on increasing efficiency, sustainability, and animal welfare.
Dr. Giordano oversees a group of academics and students—including doctorate student Martin Perez—supporting this initiative. Focused on improving cow health and farm productivity using creative sensor technologies, Perez is crucial in creating automated monitoring systems for dairy cows. He develops fresh ideas to transform dairy farm operations and assesses commercial sensor systems.
With their team, Dr. Giordano and Perez are pushing the boundaries of dairy farming by combining innovative technology with hands-on research. Their efforts not only advance scholarly knowledge but also provide practical applications that have the potential to revolutionize the dairy sector, making it more efficient, sustainable, and animal-friendly.
Transformative Innovations in Dairy Farming: Martin Perez’s Groundbreaking Research
Modern dairy farming is changing due to Martin Perez’s pioneering efforts in creating automated monitoring systems for dairy cows. Perez promotes ongoing cow health monitoring by combining sophisticated sensors and machine learning, improving cow well-being, farm efficiency, and sustainability.
Perez uses multi-functional sensors to track rumination, activity, body temperature, and eating behavior. Using machine learning models, data analysis enables early identification of possible health problems, guaranteeing timely treatment of diseases like mastitis and enhancing cow health and milk output.
These automated devices save labor expenses by eliminating the requirement for thorough human inspections, freeing farm personnel for other chores. The accuracy of sensor data improves health evaluations and guides better management choices, thereby optimizing agricultural activities.
Healthwise, more excellent production and longer lifespans of healthier cows help lower the environmental impact of dairy operations. Practical resource usage under the direction of data-driven insights helps further support environmentally friendly dairy production methods.
Perez’s innovation is a technological advancement, a transformation of herd management, and a new agricultural benchmark. The potential of these systems to promote sustainability, increase efficiency, and enhance animal welfare is a significant turning point for the future of dairy farming, offering hope for a more advanced and sustainable industry.
Automated Health Monitoring in Dairy: Challenging the Norms of Traditional Veterinary Practices
Martin Perez and colleagues evaluated the accuracy of automated cow monitoring systems in identifying mastitis and other diseases in a rigorous randomized experiment. Two groups of cows were formed: one had thorough manual health inspections, and the other was under modern sensor monitoring. This careful design helped to make a strong comparison between creative automation and conventional inspection possible.
The results were shocking. Performance measures were statistically identical between groups under human inspection and sensor-monitored cow health. This implies that automated sensors equal or exceed human inspectors in spotting early symptoms of diseases like mastitis.
These sensors, designed for everyday farm usage, continuously monitor cow health without causing stress. Early intervention from these systems can lead to increased milk output, improved cow health, and significant cost savings, revolutionizing dairy farming practices.
These findings are noteworthy. They suggest a day when dairy farms will use technology to improve animal health and output while lowering worker requirements. While Perez and his colleagues improve these sensors, predictive analytics and preventive treatment on commercial crops seem exciting and almost here.
Harnessing Advanced Sensor Integration: A Paradigm Shift in Dairy Health Monitoring
Perez’s creative technique revolves mainly around combining many sensor data. He holistically sees cow health and production by merging sensor information tracking rumination, activity, body temperature, and eating behavior. Advanced machine learning systems then examine this data, spotting trends that would be overlooked with conventional approaches.
The real-world consequences of Perez’s technology are significant. Machine learning’s early identification of problems increases the accuracy of health monitoring and enables preventative actions. This proactive method improves cows’ health and well-being and raises the efficiency and sustainability of dairy production. The practical use and transforming power of these sensor systems in contemporary agriculture are inspiring, showing the potential for a more efficient and sustainable industry.
Propelling Dairy Farming into the Future: Perez’s Vision for Proactive Health Management with Early Sensor Alerts
Perez’s work employing early sensor alarms for preventive treatments is poised to transform dairy health management. Combining real-time sensor data on rumination, activity, temperature, and eating behavior, Perez’s systems seek to forecast health problems before they become major. This proactive strategy may revolutionize dairy farming.
Early identification may help lower diseases like mastitis by allowing quick treatments, better animal comfort, milk production maintenance, and reduced veterinary expenses. Greater agricultural profitability and efficiency follow.
Perez’s data-driven approach to decision-making draws attention to a change toward precision dairy production. Using integrated sensor data analysis, machine learning algorithms improve diagnostic and treatment accuracy, boosting industry standards. Adoption among dairy producers is projected to rise as technologies show cost-effectiveness, hence launching a new phase of sustainable dairy production.
Expanding Horizons: Revolutionizing Reproductive Management and Nutrition in Dairy Farming
All fundamental to CAST’s objectives, the innovation at CAST spans health monitoring into reproductive status monitoring, breeding assistance, and nutrition management. Researchers use semi-automated and automated techniques to change these essential aspects of dairy production. These instruments improve breeding choices using rapid data-driven insights and offer continual, accurate reproductive state evaluations.
CAST also emphasizes besting nutrition and feeding practices. This entails using thorough data analysis to create regimens combining feed consumption with cow reactions to dietary changes. The aim is to provide customized diets that satisfy nutritional requirements and increase output and health. Essential are automated monitoring systems, which offer real-time data to flexible feeding plans and balance between cost-effectiveness and nutritional value.
CAST’s reproductive and nutrition control programs are dedicated to combining data analytics and technology with conventional methods. This promises a day when dairy production will be more sustainable, efficient, tuned to animal welfare, and less wasteful.
The Bottom Line
Leading contemporary agriculture, the Cornell Agricultural Systems Testbed and Demonstration Site (CAST) is revolutionizing dairy production using technological creativity. Under the direction of experts like Dr. Julio Giordano and Martin Perez, anchored at Cornell University, CAST pushes the digital revolution in dairy production from all directions. Perez’s assessments of machine learning algorithms and automated cow monitoring systems foretell health problems with accuracy and effectiveness. While improving animal welfare and agricultural efficiency, these instruments either equal or exceed conventional approaches. Effective identification of diseases like mastitis by automated sensors exposes scalable and reasonably priced agrarian methods. Data-driven insights make preemptive management of animal health and resources possible. As CAST pushes dairy farming limits, stakeholders are urged to reconsider food production and animal welfare. From study to reality, translating these developments calls for cooperation across government, business, and academia, as well as funding. Accepting these changes will help us to design a technologically developed and ecologically friendly future.
Key Takeaways:
- The Cornell Agricultural Systems Testbed and Demonstration Site (CAST) is spearheading the digital transformation of dairy farming, focusing on cattle sensors, predictive analytics, autonomous equipment, environmental monitoring, data integration, and traceability.
- The project spans three locations in New York: the Cornell University Ruminant Center in Harford, the Musgrave Research Farm in Aurora, and the Cornell Teaching Dairy Barn in Ithaca.
- Dr. Julio Giordano, associate professor of animal science at Cornell, leads the initiative, with doctoral student Martin Perez conducting groundbreaking research on automated monitoring systems to enhance cow health, farm efficiency, and sustainability.
- Perez’s research has shown that automated sensors can be as effective as intensive manual checks in detecting health conditions like mastitis, ensuring timely treatment without negatively impacting the cows.
- Advanced sensor integration combines various data streams, such as rumination, activity, body temperature, and feeding behavior, analyzed through machine learning to identify health issues early on.
- Future goals include leveraging early sensor alerts for preventative treatments and optimizing reproductive and nutritional management through automated tools and data-driven strategies.
Summary:
Cornell University’s CAST for the Farm of the Future project is a collaboration between advanced technology and traditional agricultural methods to modernize dairy farming. Dr. Julio Giordano leads the initiative, which uses environmental monitoring, predictive analytics, autonomous vehicles, and livestock sensors to detect diseases, enhance cow health, and increase farm efficiency. The automated systems are evaluated using machine learning algorithms for proactive health management. Other CAST efforts promote optimal nutrition, feeding, and reproductive surveillance. The project gains from three New York locations: Harford, Aurora, and Ithaca. Dr. Julio Giordano is driving the integration of cutting-edge technologies into dairy production, focusing on increasing efficiency, sustainability, and animal welfare. Dr. Martin Perez is crucial in creating automated monitoring systems for dairy cows, improving cow well-being, farm efficiency, and sustainability. These devices use multi-functional sensors to track rumination, activity, body temperature, and eating behavior, enabling early identification of health problems and enhancing cow health and milk output. Perez’s data-driven approach to decision-making highlights a shift towards precision dairy production, using integrated sensor data analysis and machine learning algorithms to improve diagnostic and treatment accuracy.